Clinical Trial Data Visualization: Turning Complexity Into Clarity
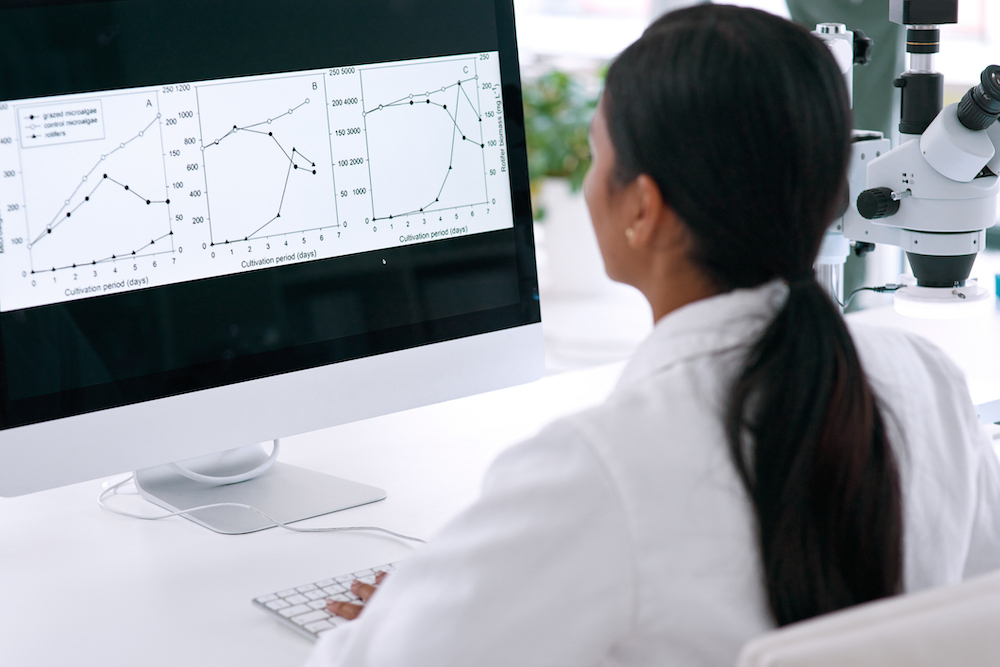
Clinical trials generate vast amounts of data, from patient demographics and laboratory results to device-captured metrics and adverse event reports. As trials increase in complexity, interpreting this data quickly and accurately has become essential.
That’s where clinical trial data visualization comes in.
By turning raw data into visual formats, research teams more easily recognize patterns, detect anomalies, and can share findings across teams. Effective visualization does more than streamline decision-making—it improves trial oversight, enhances patient safety, and supports regulatory compliance.
Why Clinical Trial Data Visualization Matters
Simplifying Complex, Multi-Source Data
Clinical trials today rely on a wide range of digital tools: electronic data capture, patient wearables, ePROs, and more. That diversity helps improve data quality, but it also makes it harder to see the big picture without getting lost in the details.
When all of this data is flowing in asynchronously, how do you track what’s working—and where your study might be falling off course?
Example: In a diabetes trial evaluating a new GLP-1 receptor agonist, participants are using continuous glucose monitors (CGMs), completing ePROs on side effects, and attending regular blood draws. Without visualization, researchers would have to manually compare CGM data with fasting glucose labs and subjective side effect reporting. Data visualization techniques like a centralized dashboard allow the study team to overlay lab results with real-time wearable data and eDiary entries—making it easier to correlate glycemic changes with reported nausea or appetite suppression.
Detecting Safety Signals Earlier
Safety concerns don’t always present in obvious ways. Often, the early signs are subtle—outliers in a lab value, an uptick in specific adverse events, or patterns that get lost in raw spreadsheets. Data visualization helps safety teams identify these patterns before they become full-blown problems.
Example: In a global vaccine trial, site-level reporting appeared normal. But when safety reviewers used a heat map to visualize adverse event reports by age and geography, they noticed a cluster of post-dose syncope events among participants over 70 at a few North American sites. This triggered an investigation that led to improved post-vaccination observation procedures for elderly participants, preventing further incidents and minimizing protocol risk.
Improving Trial Oversight and Site Management
Managing a multi-site clinical trial means keeping tabs on many moving parts: enrollment pacing, protocol deviations, screen failure rates, and data entry timelines. Without a clear view into these activities, sponsors and CROs may not intervene until problems escalate.
Visualization brings these issues to the surface in time to act.
Example: In a COPD trial testing a new inhaled triple therapy, a sponsor dashboard showed one site enrolling at twice the rate of others—initially seen as a positive. But visual tracking of spirometry results revealed that this site’s participants were consistently outside the expected range for moderate-to-severe disease. Closer review uncovered misinterpretation of inclusion criteria. Thanks to the dashboard, enrollment at the site was paused, and retraining was issued before the error could impact trial integrity.
Boosting Patient Retention and Engagement
Patient drop-off, or drop-outs, is one of the most common reasons trials fall behind schedule. But often, retention issues are predictable—they start with missed visits, incomplete diaries, or a drop in engagement. Visualization tools help study teams spot these trends early, and step in before participants disappear.
Example: In a trial evaluating a new topical JAK inhibitor for alopecia areata, participants were asked to complete regular scalp photography and symptom diaries through an app. A visualization tool flagged a steady decline in compliance from one participant over two weeks. Coordinators reached out and learned the participant didn’t feel confident using the app’s camera tool. A 10-minute walkthrough over the phone solved the issue—and likely saved the participant from dropping out entirely.
Ensuring Data Integrity and Protocol Compliance
With high-stakes endpoints—like PK sampling, imaging windows, or titrated dosing—accuracy matters. Even small deviations can jeopardize data validity. Visualization tools can let you track real-time protocol compliance, helping you catch mistakes before they spread.
Example: In a study of a new long-acting insulin, PK blood draws had to occur at precise intervals after dosing. A visual compliance tracker showed multiple deviations at a single site, where samples were being drawn 45 minutes late. With a traditional review process, this might have gone unnoticed until database lock. With visualization, the issue was flagged and corrected after just two patients.
Facilitating Better Communication Across Stakeholders
Clinical research involves many different players—sites, sponsors, CROs, safety monitors, regulators—and they don’t all need the same level of detail. Visualization helps tailor the right story for each audience, whether that’s granular patient data or high-level outcomes.
Example: As part of a pre-planned interim analysis in a COPD trial, the sponsor prepared a data visualization summary comparing exacerbation rates between treatment arms. Visual breakdowns by smoking status and eosinophil count revealed clear efficacy among participants with higher eosinophil levels. That insight led to protocol amendments aimed at targeting that responder subgroup in the next phase of enrollment—without waiting for final unblinded results.
Common Visualization Techniques for Clinical Trial Data
Different types of visualizations serve different purposes. Common tools include:
- Line Graphs – Track changes in a continuous variable over time—ideal for viewing individual or group-level trends.
- Bar Charts & Histograms – Compare counts or distributions of categorical data like adverse events, demographics, or protocol deviations.
- Scatter Plots – Visualize relationships between two variables—useful for uncovering correlations and potential causality.
- Heat Maps – Highlight patterns or outliers in large, matrix-like datasets—often used for lab values, adverse events, or site performance metrics.
- Interactive Dashboards – Aggregate data from multiple sources into a dynamic interface that updates continuously as new data is entered.
- Geospatial Maps – Visualize geographic trends in enrollment, protocol deviations, or patient outcomes across study sites.
Collectively, these clinical data visualization techniques help reduce cognitive load by distilling complex, multidimensional data into digestible insights. They allow stakeholders to focus on what’s most important without having to dig through raw datasets. This accelerates decision-making, improves data quality, and helps keep trials stay on track.
How Clinical Trial Data Visualization Is Changing
Artificial intelligence (AI) and machine learning (ML) are driving fundamental shifts in how clinical trial data is visualized, interpreted, and acted upon. Rather than simply reporting what’s happened, AI-enhanced visualization tools now offer the ability to predict, personalize, and prioritize decisions.
Here’s how AI and ML are refining the future of clinical trial data visualization:
From Retrospective to Predictive Insights
Traditional visualizations help teams understand trends after they’ve occurred. AI takes that a step further by identifying patterns that forecast what’s likely to happen next—before a critical event occurs.
Consider this: Could a participant’s dwindling ePRO engagement, minor lab changes, and irregular wearable readings point to an imminent dropout or adverse event? An ML model trained on prior trial data could flag this risk early, prompting a check-in or intervention before the problem escalates.
This kind of predictive visualization transforms how study teams manage retention, adherence, and participant safety in real time.
Enhancing Signal Detection in Complex Datasets
AI excels at pattern recognition across large, noisy datasets—especially when signals are subtle or cross multiple domains. By embedding AI into visualization tools, trial teams can detect emerging trends faster and with greater confidence.
Consider this: In a COPD trial, subtle correlations between geographic region, baseline eosinophil levels, and increased exacerbation risk might go unnoticed using standard visual tools. An AI-enhanced dashboard could surface this connection early—informing stratification strategies, site management, or adaptive protocol decisions.
Prioritizing What Matters Most
One of the biggest challenges in clinical trials today is data volume. Trial teams are inundated with information. AI helps prioritize what deserves attention and when.
Consider this: Instead of reviewing every adverse event log, what if your dashboard surfaced the top five safety signals most likely to affect participant outcomes, based on historical context and current trends? AI can filter and score events by relevance, so investigators and monitors spend their time where it matters most.
This approach reduces noise and improves the efficiency of safety reviews and operational oversight.
Supporting Personalized Visualizations
Not all trial stakeholders need the same view. Sponsors want high-level summaries. Investigators need patient-level detail. Regulators want traceability. AI can tailor data outputs based on a user’s role and preferences.
Consider this: What if a principal investigator logging into the system saw a dashboard pre-configured to highlight only their site’s protocol deviations, lab alerts, and dosing compliance—all automatically prioritized by urgency? At the same time, a sponsor might see a real-time portfolio view across multiple sites or studies.
AI-based personalization helps teams act faster, with less data overwhelm.
Automating Anomaly Detection
In complex or high-throughput studies, it’s easy for protocol deviations or data entry errors to slip through the cracks. AI can monitor for inconsistencies or outliers that suggest something isn’t right, whether it’s related to dosing errors, time stamp irregularities, or unexpected lab shifts.
Consider this: An AI-powered visualization layer could alert monitors to a site where every participant’s blood pressure reading is identical—suggesting potential data entry shortcuts or device calibration issues. These red flags allow for early intervention and protect overall data integrity.
Putting Data to Work—With the Right Site Partner
Even the most advanced data systems are only as effective as the teams interpreting and acting on them.
At Remington-Davis, we understand that successful trials depend on more than just good data—they depend on actionable insights, fast response times, and site teams that know how to translate information into interventions. Our experienced staff works seamlessly within sponsor and CRO visualization frameworks, helping identify protocol issues, safety concerns, and retention risks before they jeopardize outcomes.
Contact Remington-Davis to learn how we support clinical trials with precision, integrity, and data-driven performance.
Frequently Asked Questions
How can data visualization improve collaboration between sites, sponsors, and CROs?
Visualization makes it easier to present data in a shared, digestible format. Stakeholders can view dashboards that reflect current performance metrics across locations and offer immediate insights. When built on strong data integration, these dashboards allow teams to track enrollment, deviations, and safety signals in near real-time—creating a common view of all the data that enhances alignment and reduces back-and-forth communication.
What are some common mistakes to avoid when implementing data visualization in a clinical trial?
A few pitfalls include trying to visualize incomplete or inconsistent datasets, not validating data integration between systems, and designing dashboards that overwhelm users with too much information. It’s also common to focus on visuals without defining the questions you’re trying to answer.
Make sure your team agrees on the KPIs you want to monitor, confirm data cleanliness before launch, and test whether the tool is truly pulling all the data needed. Good visualization doesn’t just look sharp—it supports timely, informed decisions.
Can visualizations be used in regulatory submissions or audits?
Yes, when built correctly. Regulators increasingly expect transparency and traceability in how clinical data is managed. Visualizations help present data trends clearly, just make sure your data aggregation methods are documented and any visual tools meet regulatory expectations for audit trails and data provenance. A well-designed visual report can often say more than a hundred pages of tables.